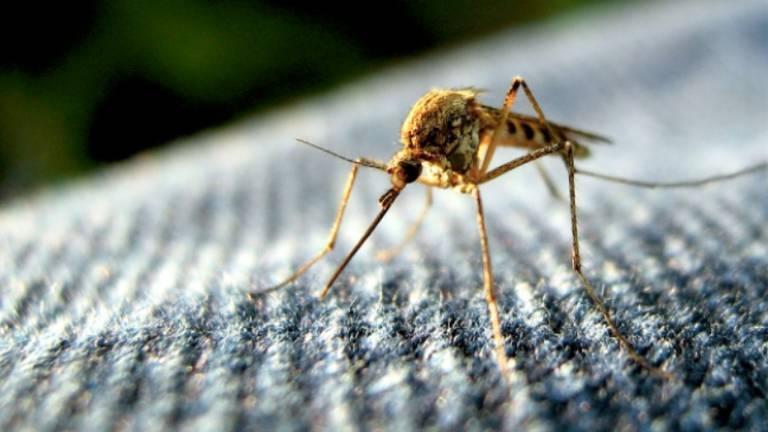
By Oliver Bent
Bed nets, insecticides and repellents are all effective intervention strategies to control the spread of malaria, but with a continuously dwindling budget, how can public officials and policymakers know what to use – where and when – to be most effective?
Malaria is caused by parasites transmitted to people through the bites of infected mosquitoes. While it’s endemic in sub-Saharan Africa (SSA), nearly half of the world’s population is at risk.
According to the World Health Organization, in 2015, there were roughly 212 million malaria cases and an estimated 429,000 malaria deaths.
Malaria control measures have led to a 29 percent reduction in mortality rates since 2010.
But I’d personally like to see this statistic eradicated, and the tools of machine learning (ML) – and more recently artificial intelligence (AI) – may provide some potential answers.
Incorporating machine intelligence
Recently at the Innovative Applications for Artificial Intelligence (AAAI-IAAI) conference located in New Orleans, I was privileged to present our recent work Novel Exploration Techniques (NETs) for Malaria Policy Interventions.
Conducted in collaboration between IBM Research – Africa and the University of Oxford – we have begun to use machine intelligence to augment the decision-making abilities of officials and explore more effective malaria policy interventions.
Our work proposes the use of different AI algorithms or agents to determine the most effective intervention strategies for specific locations.
This was made possible by publicly available research and models (OpenMalaria). The OpenMalaria simulation model can be used by computational agents to explore what-if scenarios and learn new, more effective policies for the control of the disease.
Our approach applies multiple AI agents to determine more effective malaria policies based on long-lasting, insecticide-treated nets and performing indoor residual spraying programs. We ran these computational experiments to simulate a region in Western Kenya during a five-year intervention period in the future.
Using a combination of advanced computer infrastructure and AI, we can demonstrate that it’s possible to determine higher performing future intervention strategies for a particular region.
This is important because as with anything, people find it difficult to deal with vast strategy spaces, and sometimes our decisions can be prejudiced from past experience, lack of time or influence.
We’ve developed the AI agents as “expert advisers” to help support policymakers looking for more cost-effective intervention strategies.
Combating cost
The cost effectiveness of malaria intervention strategies is significant: Given finite, distributed and uncertain investment in the elimination of malaria, AI may be able to level the playing field and coordinate how we can do the most good for the greatest number of people.
For example, a 2017 study reported that insecticide-treated nets are consistently the most cost-effective intervention across a range of transmission locations.
Yet, our results suggest that for simulated scenarios in Western Kenya, it may be more cost effective to perform indoor residual spraying programs for a small proportion of households, instead of continuing to scale the deployment of insecticide-treated nets.
We have treated the particular challenge of determining the most cost-effective malaria interventions as a stochastic multi-armed bandit problem.
Since we assume any strategy is to be implemented five years into the future, this reduces the problem to identifying which single policy as a combination of interventions is most cost effective.
While exploring novel, high-performing policies, we wish to reduce uncertainty given the stochasticity in the results supporting decisions.
Therefore, so-called Bandit algorithms, which take uncertainty into account, were a natural fit – as well as giving comparison with other techniques including Genetic Algorithms and Policy-Gradients from Reinforcement Learning.
Scratching the surface
While we have only just started to scratch the surface of artificial intelligence, we’re continuing to research how we can achieve more nuanced decisions through training machines to understand the impacts of seasonality; explore more intervention options; target interventions to specific populations; deploy across locations; and account for dynamic changes in the environment as we observe new real-world data.
I believe this would bring us closer to the objective of malaria eradication with AI.
Luckily though, we don’t believe we have to do this alone or in isolation. We have been working to bring this work and the tools we’ve developed to the cloud, leveraging even greater computing resources and geographical independence.
Hopefully, this will inspire other researchers to engage with the problem, too.
Oliver Bent is an IBM research scientist
This article originally appeared on the IBM THINK blog and was distributed by 3BL Media.
TriplePundit has published articles from over 1000 contributors. If you'd like to be a guest author, please get in touch!