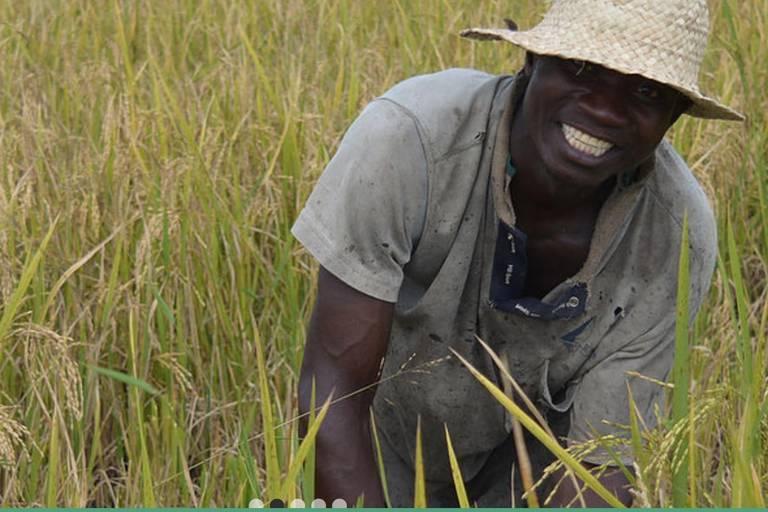
By Modern Agriculture
Arthur Samuel, an eccentric computer engineer at Stanford University, took part in what could be considered the most important game of checkers ever played. Arthur challenged the then reigning Connecticut state champion to match wits with a computer he programmed to play checkers.a
The machine emerged victorious.
Surprisingly enough, this event is not an artifact of recent history; the fateful game took place in 1961. Decades prior to the personal computer revolution, Professor Samuel built a working prototype capable of what we now call, “machine learning.” Rather than programming the 500 quintillion b potential scenarios on a checkerboard, Arthur instructed the computer to react based on games it had played in the past. After playing game after game, over time, the computer “learned” to master checkers.
Computers can develop skills without being explicitly programmed to do so.
Checkers is a game of weighing dozens of factors, calculating risk, and planning the next, most efficient move. The same principles Arthur Samuel applied to his early machine learning experiments are used today—especially in modern agriculture. One of the fastest growing areas under the discipline of “artificial intelligence” is machine learning. And this technology is being deployed across modern agriculture to create solutions with greater accuracy and at unprecedented scale.
Machine learning uses algorithms to parse data, learn from it, and make determinations without human intervention.
Millions of Choices
Plant breeders are constantly searching for a specific trait. They look for qualities to help a crop more efficiently use water, nutrients, adapt to climate change, or resist disease. For a plant to inherit a beneficial trait, researchers must find the right sequence of genes. But exactly which sequence is the right one, is a mystery in the beginning.
Plant breeders face literally millions of choices when producing a new variety.
Deep learning algorithms can take a decade of field data—insights about how crops have performed in various climates and inherited certain characteristics—and use this data to develop a probability model. With all this information, far more than any single human can grasp, machine learning can predict which genes will most likely contribute a beneficial trait to a plant. Of the millions of combinations, advanced software greatly narrows the search.
Deep learning is a subset of machine learning that can draw conclusions from various sets of raw data.
More Rigorous Testing
With the aid of machine learning, plant breeding is becoming more accurate, efficient, and capable of evaluating a much wider set of variables. Scientists can use computer simulations to conduct early tests to evaluate how a variety may perform when faced with different sub climates, soil types, weather patterns, and other factors. This digital testing does not replace physical field trials, but allows plant breeders to more accurately predict the performance of crops. By the time a new variety reaches the soil, machine learning has helped breeders create a more thoroughly vetted product than ever before.
Early Identification
When tracking any disease, early and accurate identification is essential. The traditional method of identifying plant disease is done by visual examination. This process is plagued with inefficiencies and prone to human error. For a trained computer, diagnosing plant disease is essentially pattern recognition. After sorting through hundreds of thousands of photos of diseased plants, a machine learning algorithm can spot disease type, severity, and in the future, may even recommend management practices to limit loss from a disease.
Machine learning in agriculture allows for more accurate disease diagnosis—all the while, helping eliminate wasted energy and resources from misdiagnoses. Farmers can upload field images taken by satellites, UAVs, land based rovers, pictures from smartphones, and use this software to diagnose and develop a management plan.
Machine learning in agriculture allows for more accurate disease diagnosis—all the while, helping eliminate wasted energy and resources from misdiagnoses. Farmers can upload, UAVs, land based rovers, pictures from smartphones, and use this software to diagnose and develop a management plan.
Adapting with Algorithms
Crop disease is a major cause of famine and food insecurity around the world.c A core objective of modern agriculture is to create seeds and crop protection products that provide relief to these global challenges. One of the many benefits of machine learning, is how this technology can make more accurate and precise improvements to a process. In plant breeding, machine learning is helping create more efficient seeds. Such advancements offer the potential to create even more adaptable, and productive seeds to better utilize our precious natural resources.
With memorable names like BOLT-LMM, FaST, and MAGIC,d these machine learning algorithms evoke a sense of wonder and awe.
The Accessibility of Machine Learning
What used to be reserved for major institutions is now within reach for surprisingly small, but capable teams. Similar to changes brought by access to less expensive servers and microprocessors, small startups using machine learning are beginning to reshape the industry of modern agriculture. A $5,000 supercomputer and a few ambitious computer engineers could theoretically create a huge breakthrough in plant breeding. Just a decade ago, this notion was fantasy.
The Promise of Machine Learning
Much like software, improvements in machine learning have seemingly endless possibilities. Researchers in modern agriculture are testing their theories at greater scale and helping make more accurate, real-time predictions. Modern agriculture has the potential to discover even more ways to conserve water, use nutrients and energy more efficiently, and adapt to climate change. Who knew Arthur Samuel’s quiet game of checkers would pave the way for so many advancements in modern agriculture?
Sources
aStanford University bWired Magazine cJihen Amara, Bassem Bouaziz, and Alsayed Algergawy dThe Black Boxes of Plant Breeding
Originally published on Modern Agriculture and distributed by 3BL Media.
Photo: Modern Agriculture
TriplePundit has published articles from over 1000 contributors. If you'd like to be a guest author, please get in touch!